Lorraine Université d’Excellence
Une initiative d’ingénierie globale
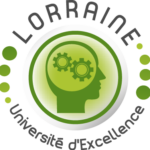
L’Initiative Lorraine Université d’Excellence (LUE), confirmée en 2021 dans le cadre des initiatives d’excellence IDEX/I-SITE, s’appuie sur une stratégie de recherche et de formation pour le site lorrain, qui identifie des domaines scientifiques partagés par les partenaires de coeur de LUE, pour développer le leadership international du site lorrain et l’ingénierie systémique autour de quelques grands défis économiques et sociétaux.
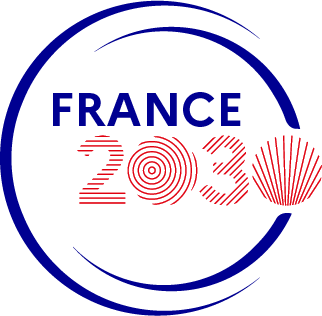
Les partenaires de l’Initiative Lorraine Université d’Excellence (LUE)
Actualités
- DrEAM – Un nouvel épisode est lancé !!!Un nouvel épisode du dispositif DrEAM (Doctor, Explore and Achieve More!) est lancé !!! Vous pouvez déposer une demande d’aide à la mobilité internationale dans le cadre de votre doctorat à l’Université de Lorraine jusqu’au 10 juin 2024 inclus. Cet … Lire plus
- Nouvelle journée de co-construction sur la notion d’habitabilité – Jeudi 4 avril 2024Dans le cadre du processus de construction du projet IMPACT EPHemeris, l’équipe porteuse du projet organise une nouvelle journée de co-construction à la présidence Brabois le jeudi 04-04-2024. Les deux dernières sessions ont été riches en discussions et ont abouti … Lire plus
- ORION – APPEL À CANDIDATURES RECHERCHE ÉCOLE THÉMATIQUE EXCELLENCE (RECOLTE)Dans le cadre de la mise en œuvre du programme ORION, l’appel à candidatures (AAC) pour le dispositif RECOLTE est ouvert pour le financement d’écoles thématiques de recherche. Ce dispositif s’adresse aux enseignants-chercheurs et chercheurs des partenaires LUE / Orion … Lire plus
Les principes d’action des programmes de l’initiative Lorraine Université d’Excellence
L’ingénierie systémique pour décloisonner les savoirs
L’interdisciplinarité comme moteur de la recherche
visibilité de la recherche de haut niveau du site lorrain
partenariats accrus avec les acteurs économiques
politique internationale mieux affirmée
Ces principes restent structurants dans la phase de pérennisation dans laquelle nous sommes maintenant. Ils sous-tendent aussi le déploiement des projets Orion et Sirius, conçus en cohérence forte avec LUE. L’interdisciplinarité reste en particulier au cœur du processus qui vise à passer d’une logique « projet » à une logique « installation pérenne de mécanismes vertueux » permettant d’impulser sur la durée des trajectoires contribuant à l’attractivité et au rayonnement du site lorrain. Ce processus doit en particulier aboutir à l’émergence de quelques grands programmes interdisciplinaires qui pourront durablement nourrir une dynamique d’excellence dont le cœur de positionnement est l’ingénierie systémique au service de défis sociétaux.
À terme, la moitié environ du budget disponible sera allouée à ces programmes interdisciplinaires. Un processus itératif est en cours, afin de faire émerger de nos communautés académiques les périmètres de ces programmes.
Les programmes récurrents
L’autre moitié du budget alimente un certain nombre de programmes récurrents dont le fonctionnement est résumé ici. À noter que certains de ces programmes seront amenés à évoluer au fil des années, suivant un processus d’autoévaluation et en s’appuyant sur les recommandations de l’Advisory Committee. Les descriptions ci-dessous correspondent donc à l’état actuel de ces programmes.
Future Leader
Candidature sur des bourses d’excellence
Widen Horizons
Immersions longues à l’international
Infra +
Mettre en réseau les infrastructures de recherche
DREAM
Mobilité internationale des doctorants
Contrats doctoraux
À l’international et avec des partenaires socio-économiques
IRP
Partenariats de recherche internationaux
Welcome@Lorraine
Accueil et accompagnement des étudiants et chercheurs
Global Incubation
Incubation
et entrepreneuriat
BOOSTER
Accompagnement des logiques de transformation
Programmes temporaires dans la phase d’émergence des programmes interdisciplinaires
En parallèle du travail d’émergence des futurs programmes interdisciplinaires, il est important de ne pas mettre un coup d’arrêt à des dynamiques collectives vertueuses, qu’elles existent de longue date ou qu’elles aient été développées dans le cadre des programmes de LUE (IMPACT, Mirabelle+, …).
Les Labex poursuivent leur programme jusqu’à leur fin programmée (2024).
Pour le reste, des appels spécifiques proposent le financement de contrats doctoraux ou post-doctoraux permettant de poursuivre les dynamiques vertueuses.
Retrouvez-nous sur les réseaux sociaux
L’INFO DU SITE ENFANT PAR
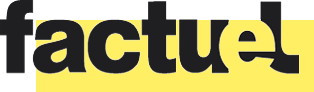